
Modern health providers have increasingly started to use artificial intelligence (AI) to reshape patient care, processes and the very fabric of the industry. Worldwide, the market size for AI in healthcare may be worth $188 billion by 2030. To paint you a picture, that is a staggering compound annual growth rate of 37% from 2022.
This demand stems from the need for efficiency and patients' evolving expectations in an era dominated by technological giants. AI in healthcare aims to amplify and augment human intelligence and interaction — not replace it.
We can already see the ripple effects of AI in healthcare. For example, AI can diagnose diseases and tailor treatments with algorithms. According to Statista, 44% of people reported a willingness to trust AI-based diagnoses and treatments in 2022.
But what else should you know about this innovative tool? Are there challenges to consider? What other AI technologies and applications can the healthcare industry use? What does the future of AI in healthcare systems look like? This guide will discuss these burning questions and more.
How Has AI Evolved in Healthcare?
Amazingly, AI's roots in healthcare trace back to the early 1970s with the creation of Mycin — an AI program designed to identify treatments for blood infections.
AI systems that supported medical progress began to appear in the 1980s and 1990s, improving surgical techniques and speeding up data processing and collection.
2000 began a revolutionary period in which AI progressed beyond biology and significantly impacted medical specializations.
AI can transform healthcare in 2024, revolutionizing treatment options, doctor-patient interactions and the industry's workforce. Though there are still concerns, AI has a bright future in healthcare because of its potential benefits.
AI is invaluable because of its remarkable ability to quickly process data. The technology is finding more healthcare applications and promising earlier and more precise diagnoses.
How Do Health Providers Use AI?
Various AI technologies and applications now provide previously unheard-of benefits in this changing market. Each is essential to promoting productivity, accuracy and patient outcomes — from improving diagnostics to streamlining administrative work.
Machine Learning
Machine learning (ML) is an effective tool for various healthcare applications because it uses statistical approaches to learn from data and adapt. ML improves diagnoses in clinical decision support by analyzing medical images, such as X-rays and MRI scans.

Pattern recognition is a technique used by ML algorithms to quickly find sequences that suggest specific disease symptoms. It allows clinicians to diagnose patients more accurately for better treatment results.
Clinical trials, therapy development and medication discovery are three other areas ML has impacted. Deep learning models analyze large-scale datasets to extract relevant information that may help develop novel medications and therapies. By examining clinical trial data on a greater scale and faster pace than ever before, ML can uncover previously unidentified side effects, which in turn boosts patient outcomes and procedure safety.
Moreover, ML technologies can cut costs by making healthcare operations more efficient and effective. Algorithms streamline processes for securing patient information and making appointments, saving time and money on labor-intensive jobs.
ML can also provide proactive monitoring by interfacing with medical equipment and automatically updating electronic health records or when a patient's status changes. This proactive approach allows health professionals to deliver accurate and timely care.
Natural Language Processing
Natural language processing (NLP) can revolutionize several areas inside the healthcare sector, most notably in clinical documentation analysis.
Maintaining medical histories, reports and patient data is essential, but manual documentation can be tedious and brings a risk of human error. NLP automates these tasks by examining free-text clinical notes, identifying vital details, classifying diagnoses and producing organized summaries. This innovation improves speed and accuracy, allowing healthcare workers to make better decisions with data analysis.
Turning spoken words into written text is an essential yet time-consuming task. Fortunately, NLP algorithms are excellent at transcribing patient-doctor exchanges and producing precise and comprehensive documentation of these conversations. This feature can advance medical documentation and save healthcare providers valuable time.
Furthermore, programmers can develop conversational AI for patient interactions thanks to NLP. Improving patient education and engagement is one of the industry's primary objectives. NLP examines text created by patients to produce customized recommendations, educational resources and personalized responses.
This individualized strategy gives patients the timely information they need to make informed choices, which may lead to better health outcomes.
Rule-Based Expert Systems
In healthcare, clinical decision support systems rely on rule-based expert systems. These help practitioners make well-informed decisions about patient care by using a predetermined set of principles.
The system applies the rules to recommend possible diagnoses or appropriate treatments when a patient shows particular symptoms. For example, through its integration with electronic health records, The California Department of Social Services ensures that medical providers make choices which follow established standards and best practices for efficient delivery.
As digital assistants, these systems help medical professionals navigate challenging clinical situations by giving them real-time insights. However, the complexity of these systems makes it more challenging to manage conflicts and guarantee accuracy.
While historically significant, rule-based expert systems are gradually giving way to more sophisticated methods like data-driven techniques and ML algorithms. This transition emphasizes dynamic adaptability and scalability in managing large and complex medical datasets, reflecting the industry's changing needs.
Healthcare providers traditionally made their decisions based on rule-based systems, but evolving approaches are leveraging the potential of data-driven intelligence to assist in making decisions, which improves patient outcomes and care further than ever before.
AI-Based Physical Robots
AI-based physical robots have become groundbreaking instruments in the rapidly changing healthcare industry, particularly in surgical settings. This technology complements human surgeons' skills and changes the nature of medical procedures. These physical robots are not simply mechanical objects — they are intelligently integrated systems that perform various tasks beyond standard automation.

Since debuting in the early 2000s, surgical robots have attracted widespread interest and acceptance. These robots have improved dexterity, precision and vision capabilities, making them invaluable assets for surgeons. Surgeons can control these robots remotely, allowing them to perform minimally invasive procedures safely and accurately.
For surgical robots, AI amplifies human abilities and knowledge in disciplines like gynecology, urology and otolaryngology. AI systems built into these robots help with real-time data analysis, picture interpretation and procedural insights — though experienced surgeons still make crucial decisions. Paired with human expertise, AI-driven robotics can produce better surgical outcomes, accelerate patient recoveries and lower patient risks.
The use of AI-driven physical robots points to a future where innovation will keep pushing the envelope of what is possible. These robots have the potential to play an increasingly significant role in medical technology as they become more sophisticated and adaptive. The union of robotics with AI forecasts a new era of opportunities in which humans and machines may work together to improve healthcare outcomes.
Robotic Process Automation
Robotic process automation (RPA) mimics human operations within information systems by automating structured digital tasks. It simplifies procedures while maintaining cost-effectiveness, transparency and ease of programming.
Automating administrative duties is one of the most valuable uses of RPA in the healthcare industry. These cover various tasks, such as managing medical records, processing claims, clinical documentation and revenue cycle management. RPA is excellent at managing routine and rule-based tasks, which can help with precision and consistency in administrative operations.
Notably, RPA makes balance billing — a challenging process with strict deadlines and notification requirements — more controllable. Incorporating RPA into the No Surprises Act compliance framework makes it easier to adhere to regulations, guaranteeing prompt replies and effective resolution.
RPA is crucial in managing reference-based pricing methodologies. RBP is a cost-effective claims pricing mechanism based on objective values for healthcare services. RPA assists in analyzing these numbers, streamlining the payment procedures and reducing the risk of overbilling.
With the support of an industry-leading A.M. top-rated reinsurance market, The Phia Group's Balance Bill SafeGuard program integrates RPA to cap payments and guarantee prompt settlements. The Phia Group provides benefit plans with unmatched security and fully supports plan participants by using RPA with legal guidance.
Moreover, RPA's flexibility includes using chatbots to improve patient interaction. Automated conversational agents streamline interactions by managing activities like prescription refills, appointment scheduling and mental health and wellness assistance. RPA-powered chatbots can enrich the patient experience while reducing the workload for medical staff.
Lastly, integrating RPA and image recognition opens new possibilities for healthcare automation. RPA efficiently enters information into transactional systems by extracting data from pictures, like faxed papers.
Other AI Technologies
Voice recognition technology (VRT) uses artificial intelligence to read spoken audio and translate it into text on a computer screen, providing a multimodal way to enhance healthcare delivery.
Reducing the requirement for written documentation is one of VRT's foremost benefits. VRT assists patients who struggle with textual communication because of illnesses or accidents. It bridges the gap for those with mobility, vision or speech issues by facilitating face-to-face contact with healthcare professionals.
Doctors also benefit from this technology's ability to transcribe spoken words into text. Combined with NLP, this convenient feature can increase productivity and help alleviate the problem of physician burnout by freeing up medical professionals' time.
Challenges of AI in Healthcare

Though AI can revolutionize the field, there are also potential drawbacks. Industry leaders must address and resolve these issues to ensure AI's ethical, safe and legal application.
Ethical Considerations
Introducing AI in healthcare has brought profound ethical considerations to light, especially in contexts where AI may supplant at least some of the decision-making traditionally done by humans. Key challenges include concerns around privacy, responsibility and openness in healthcare choices made by or in conjunction with AI.
The lack of transparency in some AI systems — particularly DL algorithms used for picture analysis — presents a serious ethical dilemma. The inability to decipher or interpret these algorithms makes it more challenging to communicate diagnoses to patients, impeding mutual understanding and trust.
Furthermore, since AI is imperfect, patient diagnosis and care errors are unavoidable. The task of determining accountability for these mistakes becomes a complex undertaking.
Another concern is that AI systems will likely handle patient data in a way that lacks human doctors' empathy for their patients. Algorithmic bias is another ethical worry, since AI systems may display or even amplify underlying prejudices based on racial or gender identity.
Data Privacy and Security Concerns
Safeguarding sensitive patient information is critical when AI is applied to healthcare. Robust data privacy and security safeguards are necessary to prevent unwanted access, security breaches and abuse of patients' most personal data.
Strict security measures are essential because healthcare systems are vulnerable to cyberattacks due to their interconnectedness – a breach in one system can easily cascade into others. A data security breach could worsen the negative impacts of AI in healthcare, eroding patient trust and confidence in these technologies.
Regulatory Compliance and Standards
Healthcare AI applications require strict industry standards and regulatory frameworks in which to operate. Implementing AI solutions presents hurdles for developers and healthcare organizations due to inconsistent standards.
Regulatory bodies must adapt quickly to keep up with the rapidly evolving AI ecosystem and craft regulations that are comprehensive, flexible and capable of addressing new challenges. Striking a balance between innovation and responsible regulation is critical to promote the proper development and application of AI technologies in healthcare.
The Future of AI in Healthcare
AI's increased integration into healthcare systems will significantly impact administrators and staff. Healthcare workers must improve and continue training to have the requisite knowledge to collaborate with AI technologies.
Though AI might automate some repetitive jobs, experts project the technology will complement human efforts rather than completely replace them. Healthcare workers may shift toward roles requiring uniquely human skills like empathy, persuasion and nuanced decision-making.
Achieving a balance between AI efficiency and human expertise will be crucial for future employment plans in healthcare.
A Look Ahead
The challenge is not whether it's possible to use AI technologies in clinical practices, but how to incorporate them into routine operations in the most effective and responsible manner.
Several essential elements — including financial assistance, standardization, regulatory approvals and clinical education — may influence widespread acceptance.
We will likely see significant developments in powerful AI algorithms in the next five to ten years. Such algorithms will be more effective, take less time to train and use unlabeled data. They may also be able to integrate various organized and unstructured health data, such as behavioral data, imaging and EHRs.
During this time, healthcare companies can move in status from adopters to co-innovators by actively contributing to creating AI systems for precision medicine.
AI systems will likely become even more intelligent in the next decade, providing precision medicine through connected care and AI-augmented healthcare. This change may potentially replace the current one-size-fits-all approach to healthcare with a more individualized, data-driven strategy for managing health and disease.
AI-enabled care should significantly improve productivity, patient satisfaction and healthcare results. In this networked care ecosystem, wearables and sensors combined with AI chatbots and virtual assistants will be essential.
Partner With The Phia Group
Incorporating AI technologies in healthcare represents a new epochal innovation, transforming all aspects of the healthcare system from patient diagnosis and treatment to administrative processes that facilitate and fund that care. Healthcare administrators and policymakers must practice strategic management to fully realize AI's potential.
Consider collaborating with The Phia Group when exploring this new and exciting terrain. With the help of our cutting-edge technologies and tactics – and our well-earned expertise in healthcare plan development, claim negotiation and balance billing settlement – we empower businesses to take charge of expenses and offer their employees the best benefits possible.
Contact us online, and we'll help you simplify the complexities of healthcare management with our cost-saving services and innovative solutions.
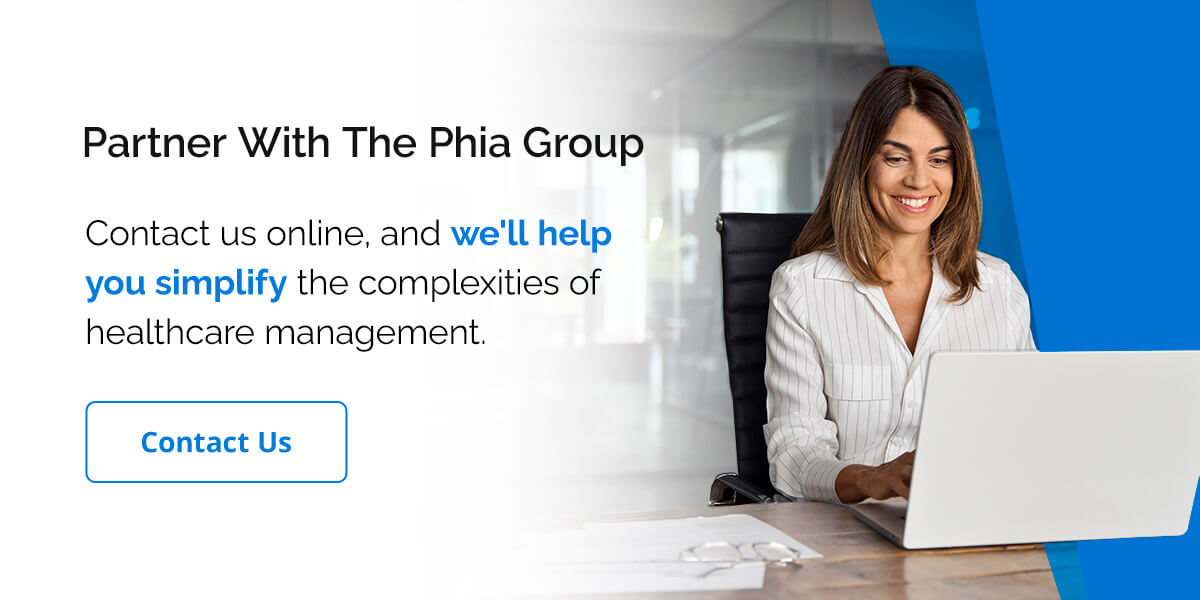